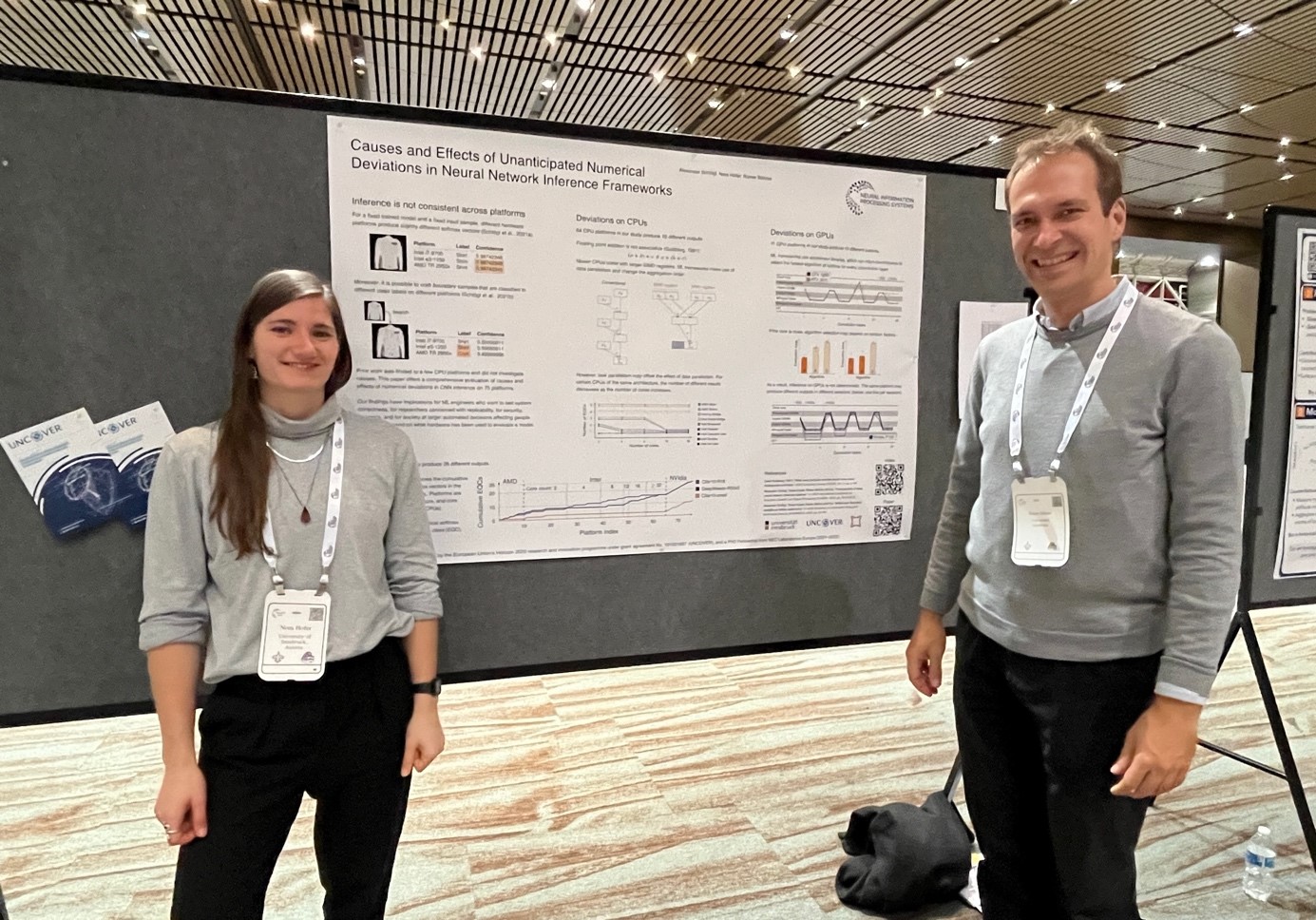
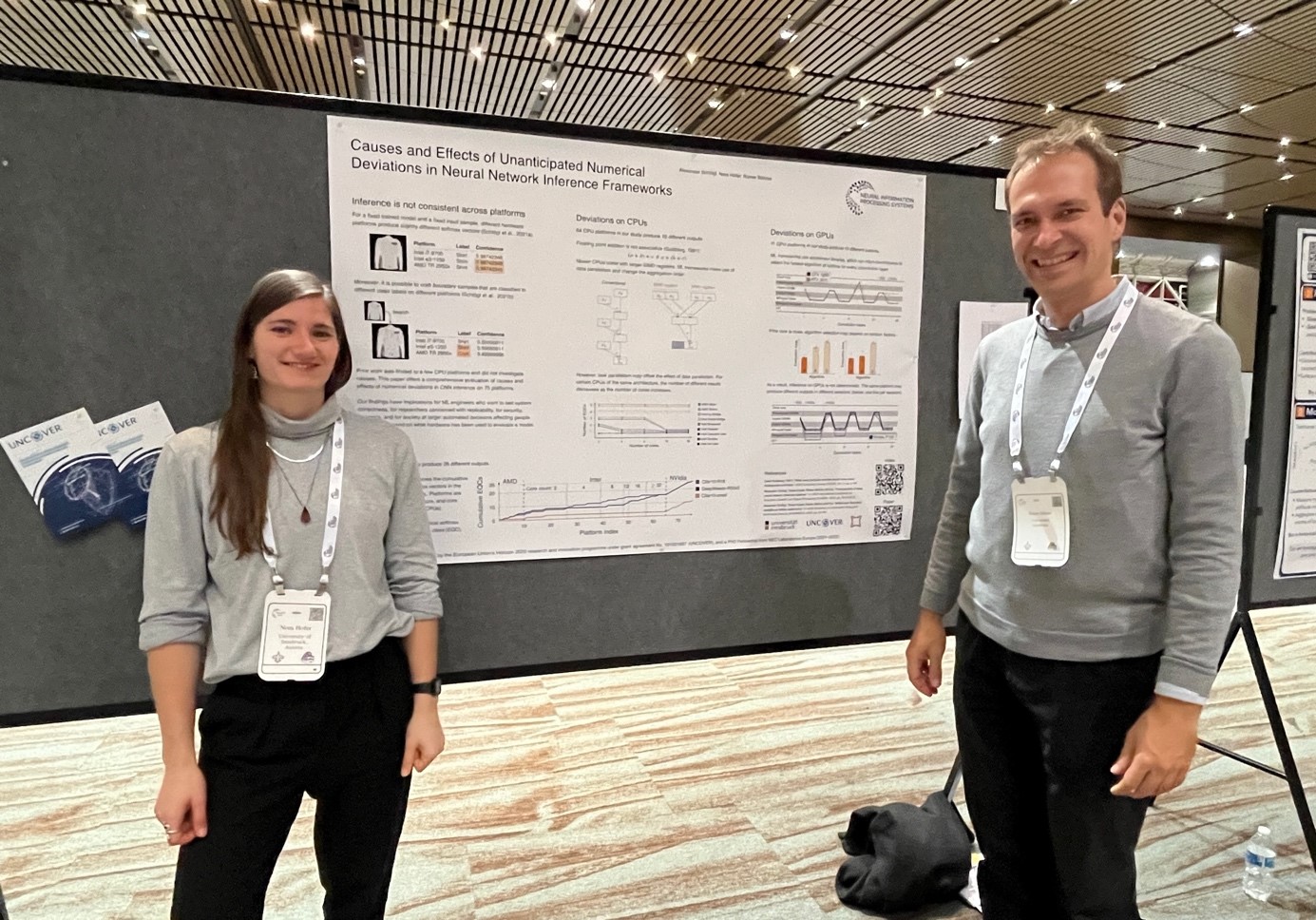
UNCOVER’s technical response to the threat of steganography relies on advanced machine learning (ML). However, state-of-the-art ML is not flawless. Classification results can be affected by various factors, such as the data, the model, and – as UNCOVER researchers point out – the hardware on which the model is executed. When ML is used for steganalysis, reliability is crucial for ensuring that investigations and legal actions are based on accurate and trustworthy evidence. Unanticipated model outputs could potentially lead to incorrect accusations or failure to detect security threats. Research by the UNCOVER project partner University of Innsbruck shows for the first time how hardware-specific optimizations can cause numerical deviations in inference results when executed on different hardware platforms. Largely overlooked by ML researchers and practitioners, this factor is so relevant that the paper was selected to be presented at one of the most competitive international conferences in machine learning.
The paper entitled “Causes and Effects of Unanticipated Numerical Deviations in Neural Network Inference Frameworks” by Alexander Schlögl, Nora Hofer and Rainer Böhme reports results for 75 different platforms, including CPUs and GPUs from different vendors and generations. It analyses causes, discusses potential mitigation strategies, and concludes that numerical deviations caused by hardware-specific optimizations are a serious and overlooked issue that can affect the reliability, security, and forensicability of ML applications.
The paper was presented at the 37th Conference on Neural Information Processing Systems (NeurIPS) in New Orleans, LA, in December 2023, and can be accessed here: https://informationsecurity.uibk.ac.at/pdfs/SHB2023_NEURIPS.pdf
A video was also made available here:
Cookie | Duration | Description |
---|---|---|
cookielawinfo-checkbox-analytics | 11 months | This cookie is set by GDPR Cookie Consent plugin. The cookie is used to store the user consent for the cookies in the category "Analytics". |
cookielawinfo-checkbox-functional | 11 months | The cookie is set by GDPR cookie consent to record the user consent for the cookies in the category "Functional". |
cookielawinfo-checkbox-necessary | 11 months | This cookie is set by GDPR Cookie Consent plugin. The cookies is used to store the user consent for the cookies in the category "Necessary". |
cookielawinfo-checkbox-others | 11 months | This cookie is set by GDPR Cookie Consent plugin. The cookie is used to store the user consent for the cookies in the category "Other. |
cookielawinfo-checkbox-performance | 11 months | This cookie is set by GDPR Cookie Consent plugin. The cookie is used to store the user consent for the cookies in the category "Performance". |
viewed_cookie_policy | 11 months | The cookie is set by the GDPR Cookie Consent plugin and is used to store whether or not user has consented to the use of cookies. It does not store any personal data. |